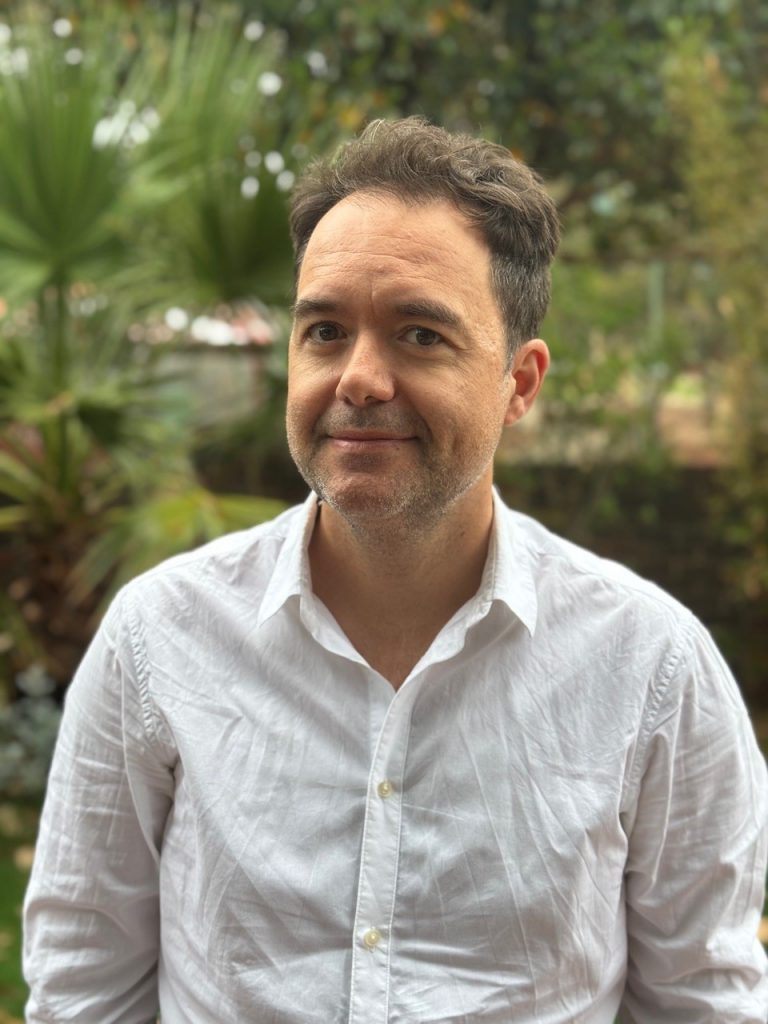
Santiago Badia
Monash University
Title: Recent advances in unfitted finite element methods
Abstract
In this talk, I will give an overview of the last advances in unfitted finite element techniques for the numerical approximation of partial differential equations. Standard finite element methods (FEMs) require cumbersome and time-consuming body-fitted mesh generation. Conversely, unfitted FEMs provide a great amount of flexibility at the geometrical discretisation step. They can embed the domain of interest in a geometrically simple background grid (usually a uniform or an adaptive Cartesian grid), which can be generated and partitioned much more efficiently. Analogously, they can easily capture embedded interfaces. As a result, unfitted FEMs are generating interest in applications with moving interfaces and varying domains. However, naive unfitted methods lead to unstable and severe ill-conditioned discrete problems, unless a specific technique mitigates the problem. Different techniques have been developed so far, which rely on perturbation (stabilisation) of the problem itself, or a redefinition of finite element spaces based on aggregation meshes and discrete extension operators. We will describe the main challenges and methods. We will show links between different approaches and their effectiveness. We will cover topics like space-time discretisations, moving interfaces, adaptive refinement and high-contrast interface problems. We will also discuss the geometrical discretisation and integration steps in the unfitted workflow. Numerical analysis results, experiments, and implementation aspects will be discussed.
Short bio
Santiago Badia is a Distinguished Professor of Computational Mathematics at Monash University (Melbourne, Australia). Previously, he served as a Professor at UPC (Barcelona, 2009–2019) and as a researcher at Sandia National Labs (Albuquerque, 2007–2008) and Politecnico di Milano (2006). His research advances finite element methods in singularly perturbed regimes, indefinite systems, multiphysics, and multiscale problems. He has made notable contributions to stabilized and unfitted finite element methods, large-scale parallel domain decomposition solvers, and coupling techniques, authoring over 100 publications in these areas. He also leads open-source scientific software projects, including Gridap. Prof. Badia has earned numerous prestigious awards, such as the 2016 Agustin de Betancourt Award (Royal Academy of Engineering, Spain), the ICREA Academia Award (Catalonia), the 2012 Young Researcher Award in Applied Mathematics (Spain), the Juan Carlos Simo Award (Spain), the 2006 ECCOMAS PhD Award (Europe), and the 2006 SEMNI Award for the best PhD Thesis in Computational Mechanics (Spain). He has led 13 major research projects across Australia, Spain, and Europe, including an ERC Starting Grant, two ERC Proof of Concept Grants, two Marie Curie Fellowships, and two ARC Discovery Projects.
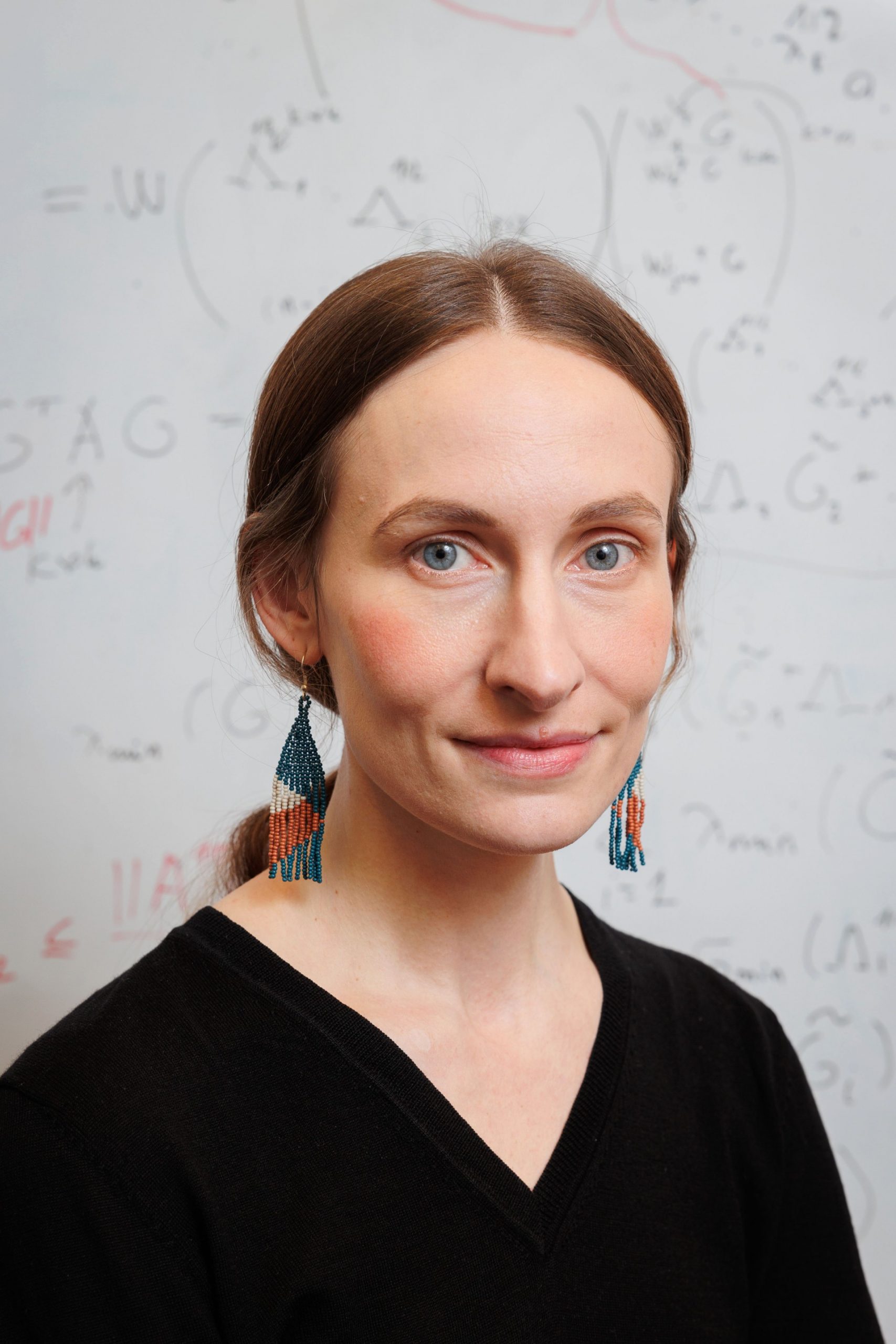
Erin Carson
Charles University
Title: Mixed Precision Matrix Computations
Abstract
Support for arithmetic in multiple precisions and number formats is becoming increasingly common in emerging architectures. Mixed precision capabilities are already included in many machines on the TOP500 list and will be a crucial hardware feature going forward. From a computational scientist’s perspective, our goal is to determine how and where we can safely exploit mixed precision computation in our codes to improve performance. This requires both an understanding of performance characteristics as well as a rigorous understanding of the theoretical behavior of algorithms in finite precision arithmetic.
We discuss the challenges of designing mixed precision algorithms and give three cases where low precision can often safely be used to improve performance. One such case, common in computational science, is when there are already other significant sources of “inexactness” present, e.g., discretization error, measurement error, or algorithmic approximation error. In this instance, analyzing the interaction of these different sources of inexactness can give insight into how the finite precision number formats should be chosen in order to “balance” the errors, potentially improving performance without a noticable decrease in accuracy. We present a few recent examples of this approach, which demonstrate the potential for the use of mixed precision in numerical linear algebra.
Short bio
Erin Carson is an Associate Professor at the Faculty of Mathematics and Physics at Charles University. Her research involves the analysis of matrix computations and the development of parallel algorithms for large-scale settings, with a particular focus on their finite precision behavior on modern heterogeneous hardware. She currently serves as Secretary of the SIAM Activity Group on Supercomputing, as the co-chair of the GAMM Activity Group on Applied andNumerical Linear Algebra, as an Associate Editor of ACM TOPC and SIAM SIMAX, and as a member of the EuroHPC Joint Undertaking Access Resource Committee. She is the recipient of the 2025 Wilkinson Prize in Numerical Analysis and Scientific Computing.
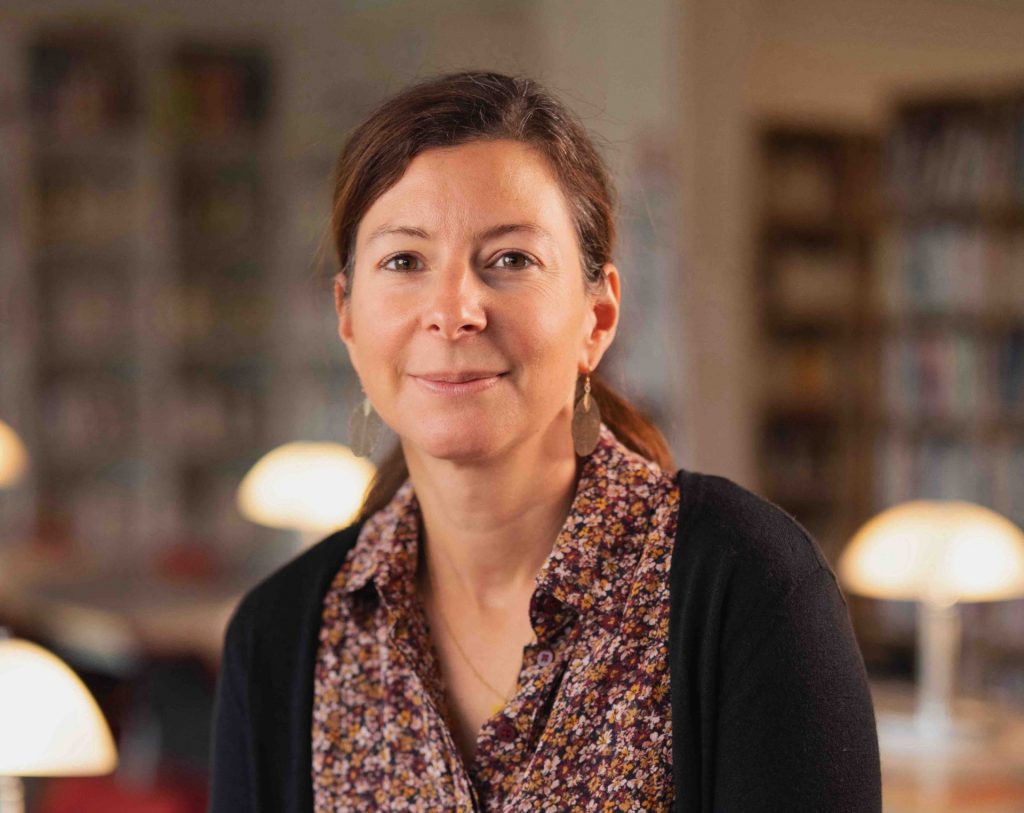
Stéphanie Chaillat
CNRS – Laboratoire POems
Title: Fast Boundary Element Methods Beyond Homogeneous Media: Towards Realistic Wave Propagation Modeling
Abstract
Boundary Element Methods (BEMs), based on the discretization of boundary integral equations, have proven particularly well-suited for modeling wave propagation in unbounded domains. In recent years, significant advances have been made within the BEM community to make these methods applicable to realistic configurations. Fast algorithms—such as the Fast Multipole Method, low-rank approximations, and mesh adaptivity—have been developed to overcome the memory limitations inherent to BEM, particularly the dense nature of the system matrix. These advances have also significantly reduced computational costs, making BEM a competitive option for large-scale simulations.
While fast BEMs are now mature and effective for problems involving homogeneous media and simple geometries, their applicability remains limited in more complex scenarios. In this talk, I will discuss two directions to extend the scope of BEM-based methods.
First, I will explore how concepts from volumetric domain decomposition methods can inspire new coupling strategies between FEM and BEM for multiphysics problems. Second, I will present recent developments based on the multi-trace formalism, inspired by domain decomposition methods, which enable the modeling of piecewise homogeneous domains.
These approaches will be illustrated through numerical results that highlight their potential for addressing challenging wave propagation problems in both academic and industrial contexts.
Short bio
Stéphanie Chaillat is a CNRS Research Director and a member of the POEMS team (a joint research unit between CNRS, INRIA, and ENSTA Paris). She received her PhD in computational mechanics from École des Ponts in 2008, followed by a postdoctoral position at the College of Computing at the Georgia Institute of Technology in Atlanta. She joined CNRS in 2010.
Her research lies in the field of numerical simulation of wave propagation, with a particular focus on the development of fast and accurate methods, especially boundary element methods (BEMs). She is interested in the modeling of realistic wave phenomena with significant scientific, industrial, and environmental impact, such as seismic waves, underwater acoustics or fluid-structure interactions. Her work combines mathematical modeling, numerical analysis, and algorithmic implementation.
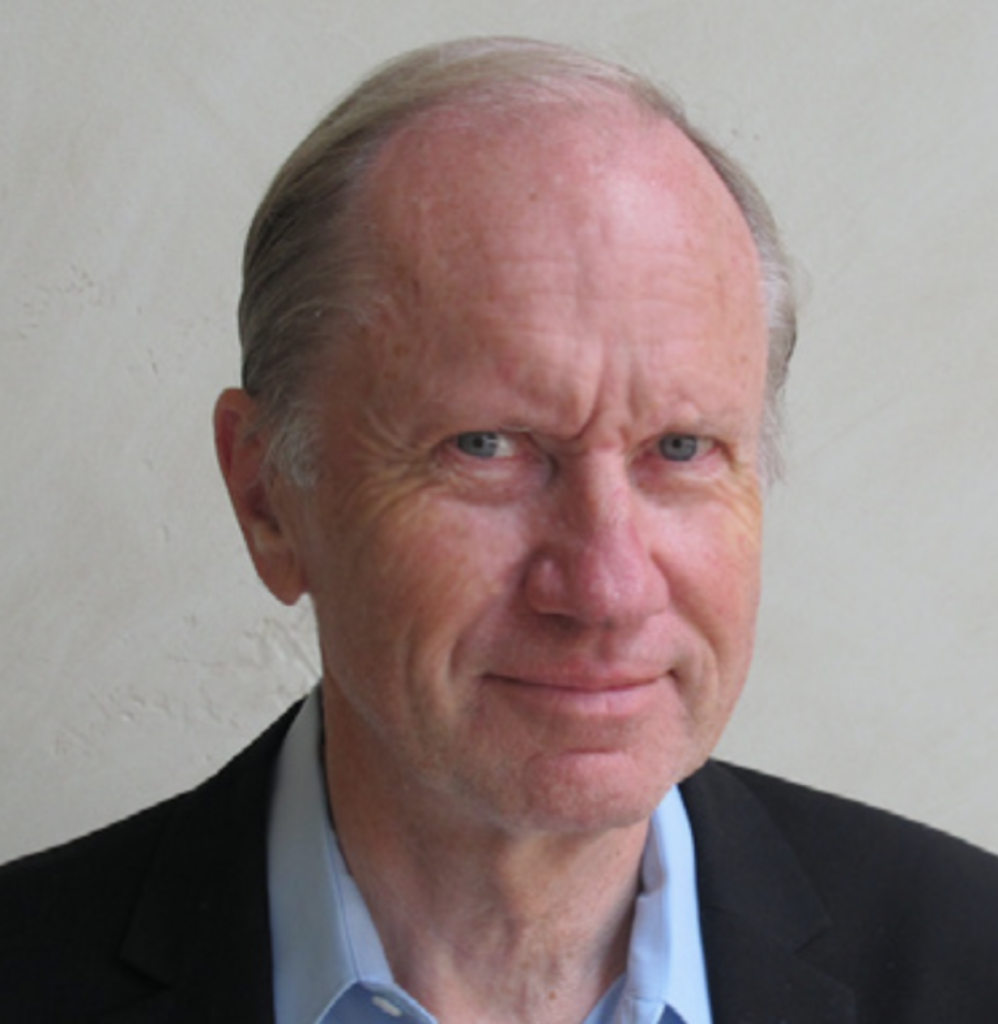
Björn Engquist
The University of Texas Austin
Title: Domain Decomposition for Molecular Dynamics
Abstract
We will first review molecular dynamics based on empirical potentials and discuss domain decomposition for distributed computing as is practiced in the computational molecular dynamics’ community. The domain decomposition is done in space or time domain but also in probability. Then we will focus on milestoning, which is a computational methodology introduced by Ron Elber. Milestoning is a domain decomposition strategy that aims at reducing the overall computational complexity. The goal is to be able to simulate processes that occur over relatively long time as, for example, protein folding. The domain boundaries are hare called milestones and the coupling is in between fluxes and sources. We assume a stochastic model and analyze the somewhat unorthodox domain coupling via the related Fokker-Planck equation.
Short bio
TBA
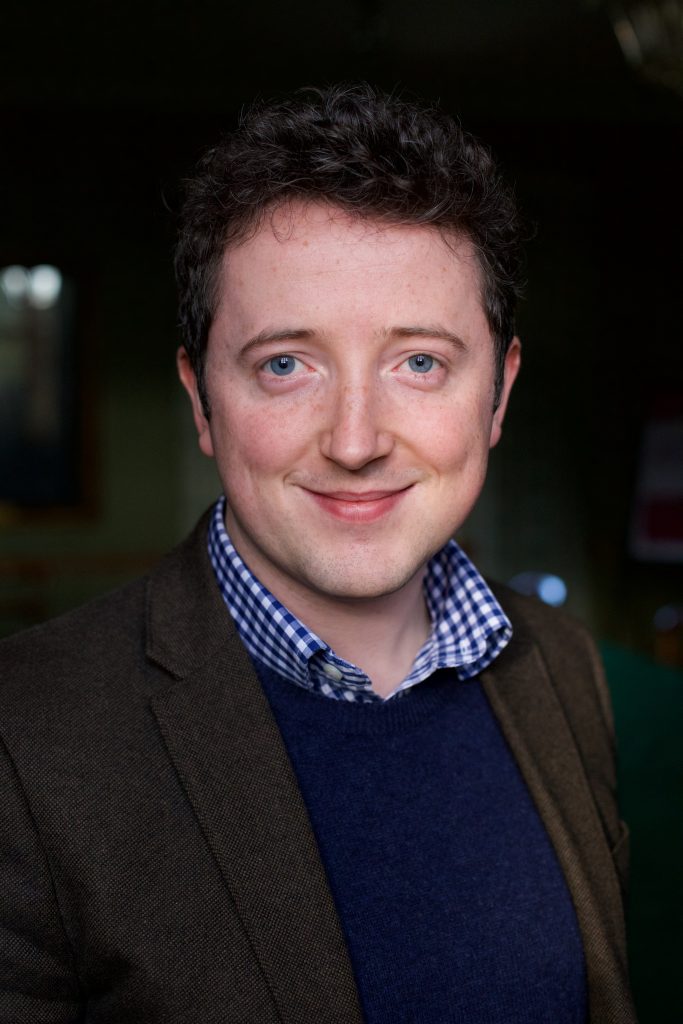
Patrick Farrell
University of Oxford
Title: Fast high-order solvers on simplices for the de Rham complex
Abstract
We present new finite elements for solving the Riesz maps of the de Rham complex on triangular and tetrahedral meshes at high order. The finite elements discretize the same spaces as usual, but with different basis functions, so that the resulting matrices have desirable properties. These properties mean that we can solve the Riesz maps to a given accuracy in a p-robust number of iterations with O(p6) flops in three dimensions, rather than the naive O(p9) flops.
The degrees of freedom build upon an idea of Demkowicz et al., and consist of integral moments on an equilateral reference simplex with respect to a numerically computed polynomial basis that is orthogonal in two different inner products. As a result, on the reference equilateral simplex, the resulting stiffness matrix has diagonal interior block, and does not couple together the interior and interface degrees of freedom. Thus, on the reference simplex, the Schur complement resulting from the elimination of interior degrees of freedom is simply the interface block itself.
This sparsity is not preserved on arbitrary cells mapped from the reference cell. Nevertheless, the interior-interface coupling is weak because it is only induced by the geometric transformation. We devise a preconditioning strategy by neglecting this interior-interface coupling. We precondition the interface Schur complement with the interface block, and simply apply point-Jacobi to precondition the interior block. The combination of this approach with a space decomposition method on vertex and edge star patches allows us to efficiently solve the canonical Riesz maps at very high order.
Authors: Patrick E. Farrell, Pablo D. Brubeck, Robert C. Kirby, Charles Parker
Short bio
Patrick Farrell is a Professor in the numerical analysis group at the University of Oxford, and (for 2025-2026) the Donatio Universitatis Carolinae Chair in the faculty of mathematics and physics at Charles University in Prague. His research interests are in the numerical solution of partial differential equations arising in physics and chemistry.
He obtained his bachelor’s degree in mathematics from the University of Galway, and his doctorate from Imperial College London in 2010. His doctoral thesis won the Roger Owen prize from the UK Association for Computational Mechanics, and the Janet Watson prize from Imperial.
He has been awarded an EPSRC Early Career Research Fellowship (2013-2018), the 2015 Wilkinson Prize for Numerical Software, second place in the 2015 Leslie Fox Prize in Numerical Analysis, the 2021 Charles Broyden Prize in optimisation, a 2021 Whitehead Prize from the London Mathematical Society, and the 2025 SIAM Germund Dahlquist Prize.
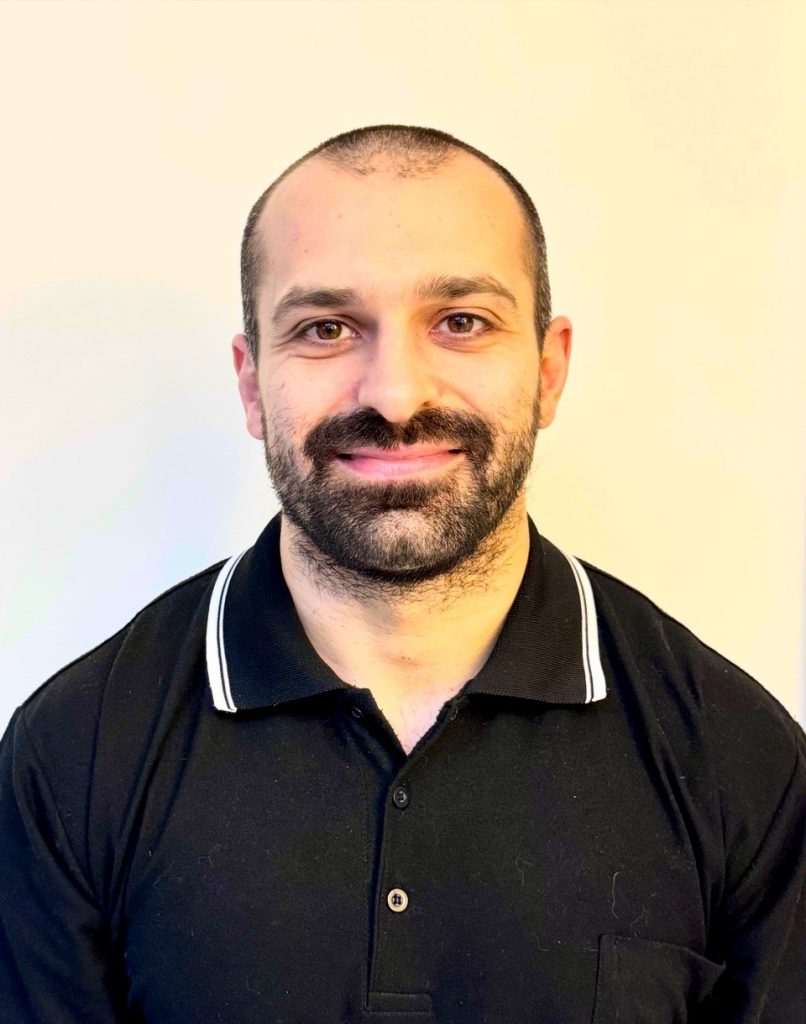
Pierre Jolivet
Sorbonne Université, CNRS, LIP6
Title: Robust overlapping Schwarz methods and their applications
Abstract
Recent advances in domain decomposition preconditioners have made it possible to handle large linear systems of increasing complexity. In this presentation, I will give some insight into how they are efficiently implemented in high-level libraries such as PETSc and HPDDM. These implementations allow domain specialists to perform large-scale analyses that were previously difficult to deal with, even with state-of-the-art solvers.
Short bio
Pierre Jolivet is a research scientist at the French National Centre for Scientific Research (CNRS) affiliated with the Laboratoire d’Informatique de Paris 6 (LIP6) at Sorbonne Université. His research focuses on high-performance computing particularly in developing fast and robust solvers for computational sciences. He is an active contributor to various open-source libraries such as PETSc, FreeFEM, and HPDDM.
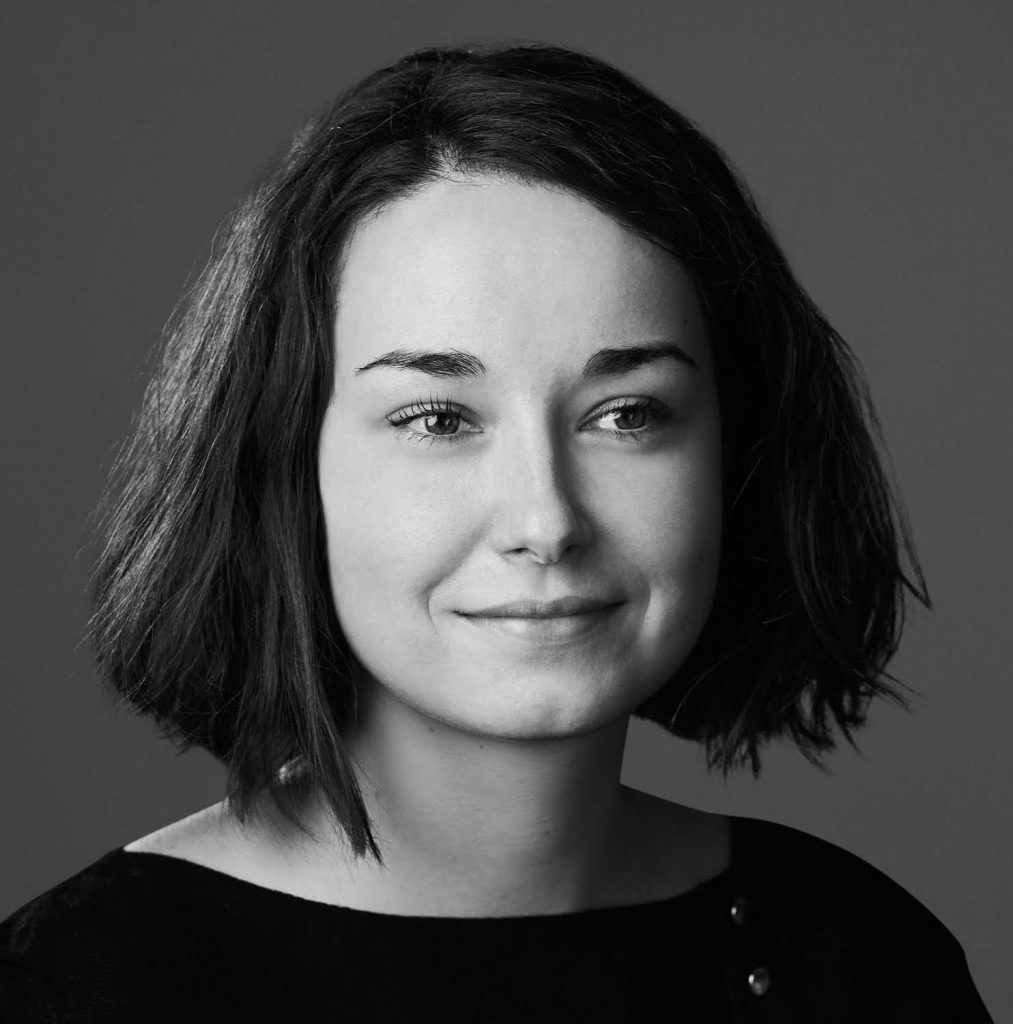
Alena Kopanicakova
University of Toulouse
Title: Training of Deep Neural Networks Using Multilevel and Domain-Decomposition Methods
Abstract
Training deep neural networks (DNNs) is predominantly carried out using stochastic gradient method and its variants. While these methods are robust and widely applicable, their convergence often deteriorates for large-scale, ill-conditioned, or stiff problems commonly encountered in scientific machine learning. This has motivated the development of more advanced training strategies that can accelerate convergence, offer better parallelism, enable convergence control, and facilitate the automatic tuning of hyperparameters. To this end, we will introduce a novel training framework for DNNs inspired by nonlinear multilevel and domain-decomposition (ML-DD) methods. Starting from deterministic ML-DD algorithms, we will discuss how to ensure convergence in the presence of the subsampling noise. Moreover, we will present several strategies for constructing a hierarchy of subspaces by exploring the properties of the network architecture, data representation, and the loss function. The numerical performance of the proposed ML-DD training algorithms will be demonstrated through a series of numerical experiments from the field of scientific machine learning, such as physics-informed neural networks and operator learning approaches.
Short bio
Alena Kopaničáková is an Associate Professor at Toulouse-INP (ENSEEIHT) and a member of the Parallel Algorithms and Optimization (APO) team at the IRIT Laboratory. She is also affiliated with the Artificial and Natural Intelligence Toulouse Institute (ANITI), where she holds an international research chair focused on the hybridization of AI and large-scale numerical simulations for engineering design. Prior to her appointment in Toulouse, she was a postdoctoral researcher at Brown University (USA) and at the Università della Svizzera italiana (Switzerland), where she also completed her PhD. Her research interests span nonlinear multilevel optimization, domain decomposition methods, scientific machine learning, hybrid (AI-augmented) iterative methods, phase-field modeling of fracture, and scientific software development.
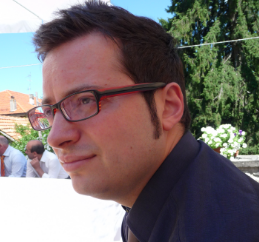
Ilario Mazzieri
Politecnico di Milano
Title: Efficient space-time methods for solving wave propagation challenges
Abstract
The numerical simulation of wave propagation presents significant challenges, particularly when dealing with complex geometries, heterogeneous media, and high-frequency regimes. Traditional time-stepping methods often struggle with achieving high-order accuracy while maintaining computational efficiency. This talk explores recent developments in domain decomposition methods for hyperbolic problems, focusing on space-time Restricted Additive Schwarz (XT-RAS) techniques. This approach provides a parallelizable framework that enhances computational performance while preserving high-order accuracy in both space and time. We analyze convergence in both continuous and discrete settings and investigate how time-windowing and time-integration schemes affect stability and performance. We then introduce pipeline and adaptive XT-RAS strategies, enabling parallelism in space and time. Numerical experiments support theoretical insights, and connections with tent-pitching approaches are discussed as a promising framework for parallel time integration.
Short bio
Ilario Mazzieri is Associate Professor of Numerical Analysis at the MOX Laboratory, Politecnico di Milano where he obtained a PhD in Mathematical Models and Methods in Engineering (cum laude, Doctor Europaeus). His research focuses on high-order numerical methods for wave propagation, including elastodynamics, acoustics, and multiphysics problems. He is the author of over 35 peer-reviewed journal articles and an invited speaker at numerous international conferences. He has coordinated and participated in many national and EU-funded research projects (PRIN, ERC, CINECA).
He is the lead developer of the seismic simulation code SPEED and co-author of the LYMPH software for polytopal methods. His work combines theoretical analysis, high-performance computing, and practical impact in geophysics and engineering.
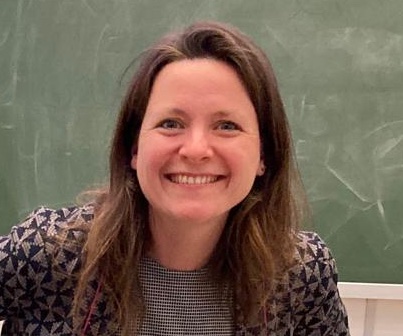
Nicole Spillane
CNRS – Ecole polytechnique
Title: Preconditioning, weighting and deflation applied to non-symmetric linear systems
Abstract
This talk considers the solution of non-symmetric linear systems by GMRES. The objective is to find out, both, how to predict the convergence of GMRES and how to accelerate it. To make a parallel with symmetric positive definite (spd) linear systems, we would like the non-spd equivalent of the statement that “a good preconditioner is a preconditioner that reduces the condition number”.
Three different accelerators are considered and combined: preconditioning, deflation, and weighting. Weighting, the lesser-known technique, consists in changing the inner product in which the GMRES algorithm operates.
In cases where the problem matrix is positive definite, it is shown that applying a symmetric preconditioner H can result in a convergence bound that depends only on how well H preconditions the symmetric part of A and on how non-symmetric the problem is. This already leads to a strategy to design scalable preconditioners by domain decomposition. Convergence is accelerated further by deflating the high-frequency vectors of a well-chosen generalized eigenvalue problem. Numerical illustrations provide backup for our findings.
Short bio
Nicole Spillane is a researcher at the French National Center for Scientific Research (CNRS). Her laboratory is the Center for Applied Mathematics of Ecole polytechnique in Paris.
As an applied mathematician, Nicole’s focus is on the analysis, development and application of large scale linear solvers. During her PhD at Université Paris Sorbonne, she participated in developing the GenEO coarse space in domain decomposition. She received two best PhD awards from AMIES and CSMA. She went on to propose the adaptive multipreconditioned conjugate gradient algorithm. In connection with this work, in 2017, she received the Leslie Fox Prize in Numerical Analysis from the UK’s Institute of Mathematics and its Applications.
Her current interests are in applying domain decomposition methods to PDEs with stochastic coefficients, and on solving non-symmetric linear systems. She is the Principal Investigator of ANR DARK on Domain Decomposition Accelerators for Robust Krylov Subspace Methods. She has also begun to explore the efficient simulation of PDEs on quantum computers.
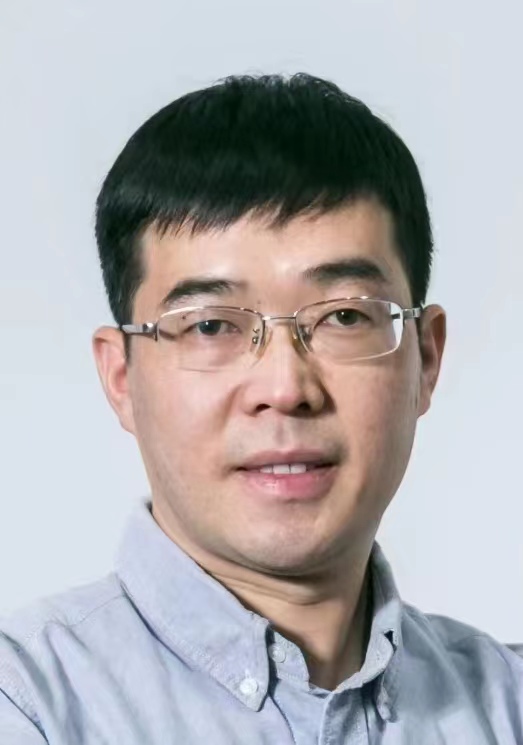
Xiaowen Xu
Institute of Applied Physics and Computational Mathematics – Beijing
Title: Divide and Conquer: Explorations in Developing Intelligent AMG Solver for Sequences of Large-Scale Sparse Linear Systems
Abstract
Solving sequences of large-scale sparse linear systems is a critical performance bottleneck in many practical applications. The primary challenge comes from the dynamically changing characteristics of sparse matrices within the sequence, making it almost impossible for any fixed algorithmic strategy to achieve optimal performance for all systems in the sequence. Given an application scenarios, how to design an intelligent solvers with automatic tuning capability has become a crucial concern in practical application, and its core is to automatically achieve the optimal mapping between matrix feature space and algorithm space. In this talk, we take the widely used AMG (algebraic multigrid) solver in practical applications as an example to introduce the exploration and practice of intelligent solvers. Based on the divide and conquer principle, using performance modeling and machine learning techniques, the AMG solver achieves automatic tuning at component-level and parameter-level. We will introduce the intelligent AMG framework and demonstrate its effectiveness in typical practical applications.
Short bio
Xiaowen Xu is a Professor and deputy of Institute of Applied Physics and Computational Mathematics (IAPCM), Beijing, China. He got his B.S degree from Xiangtan University in 2002, and his PhD degree from Chinese Academy of Engineering Physics in 2007. His research interests include high performance numerical algorithms in scientific and engineering fields, he is mainly engaged in the development of parallel sparse linear solver for large-scale numerical simulation. He is one of the core developers of the parallel programming framework called JASMIN, which enables complex numerical simulations to run efficiently on modern supercomputers and is widely used in various scientific and engineering applications in China.